By, Roberto Colom, PhD, Sherif Karama, MD, and Richard J. Haier, PhD.
Abstract.
Intelligence can be defined as a general mental ability for reasoning, problem solving, and learning.
Because of its general nature, intelligence integrates cognitive functions such as perception, attention, memory, language, or planning. On the basis of this definition, intelligence can be reliably measured by standardized tests with obtained scores predicting several broad social outcomes such as educational achievement, job performance, health, and longevity. A detailed understanding of the brain mechanisms underlying this general mental ability could provide significant individual and societal benefits. Structural and functional neuroimaging studies have generally supported a frontoparietal network relevant for intelligence. This same network has also been found to underlie cognitive functions related to perception, short-term memory storage, and language.
The distributed nature of this network and its involvement in a wide range of cognitive functions fits well with the integrative nature of intelligence. A new key phase of research is beginning to investigate how functional networks relate to structural networks, with emphasis on how distributed brain areas communicate with each other.
Human intelligence; definition, measurement, and structure.
Reasoning, problem solving, and learning are crucial facets of human intelligence. People can reason about virtually any issue, and many problems may be solved. Simple and highly complex behavioral repertoires can be learned throughout the lifespan. Importantly, there are widespread individual differences in the ability to reason, solve problems, and learn which lead to human differences in the general ability to cope with challenging situations.
These differences: (i) become more salient as the cognitive complexity of the situation becomes greater; (ii) are stable over time; and (iii) are partially mediated by genetic factors.
Various definitions of intelligence tend to converge around similar notions designed to capture the essence of this psychological factor. Jensen6 notes Carl Bereiter’s definition of intelligence: “what you use when you don’t know what to do”. After their extensive survey, Snyderman and Rothman7 underscored reasoning, problem solving, and learning as crucial for intelligence.
The “mainstream science on intelligence” report coordinated by Gottfredson highlights reasoning, planning, solving problems, thinking abstractly, comprehending complex ideas, learning quickly, and learning from experience. The American Psychological Association (APA) report on intelligence acknowledges that “individuals differ from one another in their ability to understand complex ideas, to adapt effectively to the environment, to learn from experience, to engage in various forms of reasoning, to overcome obstacles by taking thought”
Humans perceive the environment, attend to relevant stimuli, memorize episodic and semantic information, communicate, and so forth. However, these activities must be integrated in some way for: (i) adapting our behavior to the environment; (ii) selecting the most appropriate contexts; or (iii) changing the world when adaptation and selection are not an option. In our view, the integration of cognitive functions and abilities is dependent on the very general mental ability we call “general intelligence” or g for short. This integration is consistent with g as ability or as an emergent property of the brain.
Any cognitive ability refers to variations in performance on some defined class of mental or cognitive tasks (Figure 1). Abilities reflect observable differences in individuals’ performance on certain tests or tasks.
However, this performance involves the synthesis of a variety of abilities: “spatial ability,” for instance, can be regarded as an inexact concept that has no formal scientific meaning unless it refers to the structure of abilities that compose it. The problem of defining (and measuring) intelligence is the problem of defining the constructs that underlie it and of specifying their structure.
For more than a century, psychologists have developed hundreds of tests for the standardized measurement of intelligence with varying degrees of reliability and validity16 The resulting measures allowed for the organization of taxonomies identifying minor and major cognitive abilities.
J. B. Carroll, for example, proposed a threestratum theory of intelligence after the extensive reanalysis of more than 400 datasets with thousands of subjects from .almost 20 different countries around the world. Figure 2. shows a simplified depiction of the taxonomy of cognitive abilities.
This survey of factor analytic studies supports the view that intelligence has a hierarchical structure (ie, like a pyramid). There is strong evidence for a factor representing general intelligence (g) located at the apex of the hierarchy (stratum III). This g factor provides an index of the level of difficulty that an individual can handle in performing induction, reasoning, visualization, or language comprehension tests. At a lower order in the hierarchy (stratum II), several broad ability factors are distinguished: fluid intelligence, crystallized intelligence, general memory, visual perception, auditory perception, retrieval, or cognitive speed.
Lastly, stratum I is based on specific abilities, such as induction, lexical knowledge, associative memory, spatial relations, general sound discrimination, or ideational fluency.
Factor analytic surveys reveal two main findings: (i) the g factor constitutes more than half of the total common factor variance in a cognitive test or task in samples representative of the population; and (ii) various specific cognitive abilities can be identified, including the cognitive domains of language, memory, and learning, visual perception, information processing, knowledge and so forth, indicating certain generalizations of abilities; actually, there are more than 60 specific or narrow abilities. Available test batteries (a good example would be the Wechsler Adult Intelligence Scale – WAIS) measure g in addition to several cognitive abilities and specific skills. We know how to separate these influences over cognitive performance by means of statistical analyses.
There are some measures which are highly g-loaded (eg, the Vocabulary subtest of the WAIS), while others are less g-loaded (eg, the Digit Symbol Subtest of the WAIS). (Figure 3). shows how gray matter correlates become more prominent with increased g loadings of the intelligence measures. Moreover, the same measure can load differently on general and specific cognitive factors/abilities depending on the sample analyzed.
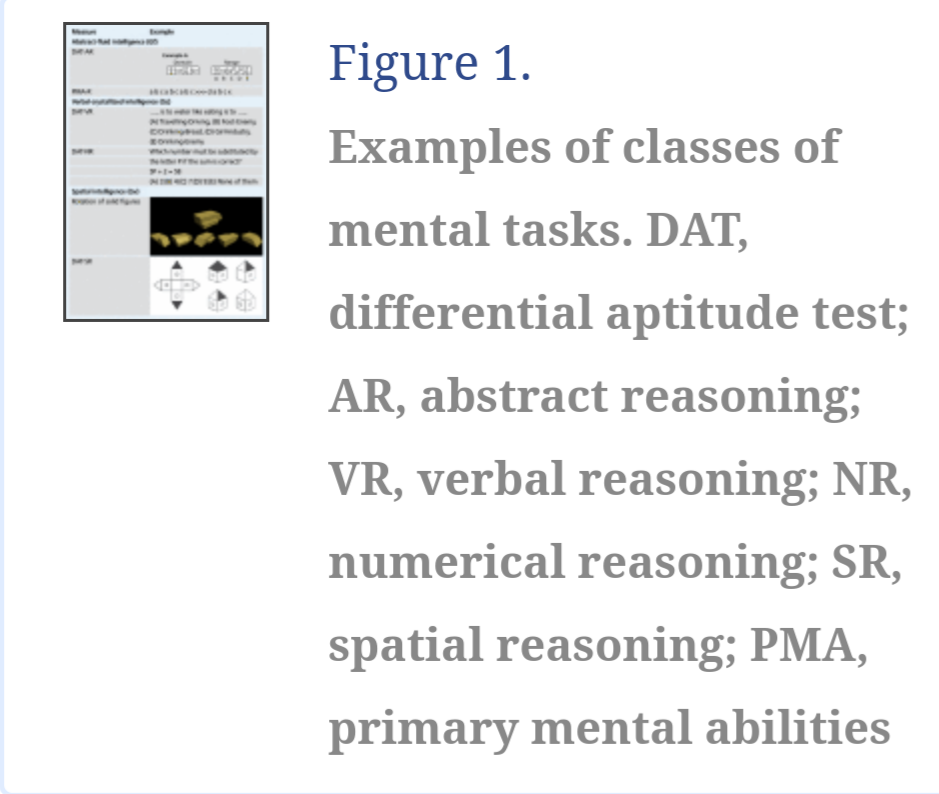
Human intelligence and the brain.
Exploring the relationships between human intelligence and the brain requires a careful consideration of the structure of human intelligence.
As evident from above, when researchers state that they are measuring intelligence by means of the Standard Progressive Matrices Test (SPM – as another example) they are telling an imprecise story because the SPM measures g plus spatial and reasoning abilities plus SPM specificity. The exact combination of these “ingredients” for the analyzed sample must be computed before saying something clear about the measured performance. This requires that studies use a battery of tests rather than just one test. Although this was not usually done for the early functional imaging studies of intelligence,21-25 it is now more common.
26-29 Results from the older and the newer studies, however, point to the importance of both whole brain and specific brain networks.
Brain size and human intelligence.
Wickett et al30 state;
“There is no longer any doubt that a larger brain predicts greater intelligence. Several research teams, using differing scan protocols, populations, and cognitive measures, have all shown that IQ and brain volume correlate at about the 0.40 level, obviously replication of this effect is no longer required. What is required now is a more fine-grained analysis of why it is that a larger brain predicts greater intelligence, and what it is about intelligence that is most directly related to brain volume” (p 1096, emphasis added).
The meta-analysis by McDaniel31 studied the relationship between in vivo brain volume and intelligence. Thirty-seven samples comprising a total of 1530 participants were considered simultaneously. These were the main findings: (i) the average correlation is 0.33; (ii) subsets of the 37 studies that allow partitioning by gender revealed that the correlation is higher for females (0.40) than for males (0.34); and (iii) the correlation does not change across age (0.33). The report concludes that these results resolve a 169-year-old debate: it is clear that intelligence and brain volumes are positively related.
Going one step further, several studies measured the volume of regions of interest (ROIs) showing the most significant correlations (controlling for total brain volumes) in frontal, parietal, and temporal brain regions, along with the hippocampus and the cerebellum.32,33 Nevertheless, regional correlations are moderate (ranging from 0.25 to 0.50) which implies that measures of total or local brain size are far from telling the whole story.
From this perspective, gray and white matter must be distinguished. In keeping with this, voxel-by-voxel (a voxel is a volume element analogous to a pixel) analyses also showed specific areas where the amount of gray and white matter was correlated with intelligence scores.24,25 The amount of gray matter is considered to reflect number and density of neuronal bodies and dendritic arborization, whereas the amount of white matter is considered to capture number and thickness of axons and their degree of myelination. Gray matter could support information processing capacity, while white matter might support the efficient flow of information in the brain.
Available reports are consistent with the statement that both gray and white matter volumes are positively related to intelligence, but that the latter relationship is somewhat greater (unweighted mean correlation values =.27 and .31 respectively).34 It is noteworthy that new studies using diffusion tensor imaging (DTI), which is the best method to date for assessing white matter, have reported DTI correlations with intelligence scores.
A distributed brain network for human intelligence.
Jung and Haier35 reviewed 37 structural and functional neuroimaging studies published between 1988 and 2007. Based on the commonalities found in their analysis, they proposed the Parieto-Frontal Integration Theory (PFIT), identifying several brain areas distributed across the brain.
These P-FIT regions support distinguishable information processing stages.
This is a summary of the proposed stages.
Occipital and temporal areas process sensory information in the first processing stage: the extrastriate cortex (Brodmann areas – BAs – 18 and 19) and the fusiform gyrus (BA 37), involved with recognition, imagery and elaboration of visual inputs, as well as Wernicke’s area (BA 22) for analysis and elaboration of syntax of auditory information.
Integration and abstraction of the sensory information by parietal BAs 39 (angular gyrus), 40 (supramarginal gyrus), and 7 (superior parietal lobule) correspond to the second processing stage.
The parietal areas interact with the frontal lobes in the third processing stage and this interaction underlies problem solving, evaluation, and hypothesis testing. Frontal BAs 6, 9, 10, 45, 46, and 47 are underscored by the model.
The anterior cingulate (BA 32) is implicated for response selection and inhibition of alternative responses, once the best solution is determined in the previous stage.
White matter, especially the arcuate fasciculus, is thought to play a critical role in reliable communication of information across the brain processing units. Nevertheless, note that the “Geschwind area” (underlying the angular gyrus) within the arcuate fasciculus may be even more important than the entire track.36
Frontal, parietal, temporal, and occipital areas are depicted.
However, Jung and Haier35 suggest that not all these areas are equally necessary in all individuals for intelligence. Discrete brain regions of the dorsolateral prefrontal cortex (BAs 9, 45, 46, and 47) and the parietal cortex (BAs 7 and 40) could be considered most important for human intelligence.
A frontoparietal network may be relevant for intelligence, but also for working memory.37 A study by Gray et al38 tested whether fluid or reasoning ability (Gf) was mediated by neural mechanisms supporting working memory. Sixty participants performed verbal and nonverbal working memory tasks. They had to indicate if a current item matched the item they saw 3 items previously (3-back). Brain activity was measured by event-related functional magnetic resonance imaging (fMRI). The demand for working memory varied across trials. Results showed that: (i) participants scoring higher on the Progressive Matrices Test (a measure related to fluid g – Gf) were more accurate in the 3-back task; and (ii) only lateral prefrontal and parietal regions mediated the correlation between Gf and 3-back performance.
These fMRI results are consistent with the voxel-based morphometry (VBM) study reported by Colom et al (N = 48).39 In agreement with the well established fact that the g factor and working memory capacity are very highly correlated,40-45 these researchers predicted that g and working memory would share significant common neural networks.
Therefore, using a VBM approach they quantified the overlap in brain areas where regional gray matter was correlated with measures of general intelligence and working memory, finding a common neuroanatomic framework supported by frontal gray matter regions belonging to BA 10 and by the right inferior parietal lobule (BA 40). Of note, this study also showed: (i) more gray matter recruitment for the more cognitively complex tasks (= more highly g loaded); and (ii) the complex span task (backward digit span) showed more gray matter overlap with the general factor of intelligence than the simple span task (forward digit span. These results were interpreted after the theory proposed by Cowan,46 namely that parietal regions support “capacity limitations,” whereas frontal areas underlie the “control of attention.”
Human intelligence and brain networks.
Roberto Colom, Universidad Autónoma de Madrid, Spain ;
Sherif Karama, McGill University, Montreal, Quebec, Canada;
Rex E. Jung, The MIND Research Network, Albuquerque, New Mexico, USA;
Richard J. Haier, University of California, Irvine, California, USA.
Dialogues Clin Neurosci. 2010 Dec; 12(4): 489–501. doi: 10.31887/DCNS.2010.12.4/rcolom
PMCID: PMC3181994